Introduction to Predictive Risk Analytics
Predictive risk analytics represents a progressive evolution within the realm of risk management. At its core, this approach utilizes advanced statistical techniques, machine learning, and artificial intelligence to forecast potential risks and their impacts on an organization’s operations, thereby enabling preemptive measures. In a world where uncertainty looms large, the integration of AI into risk management has not only transformed traditional methodologies but has also set new benchmarks for operational efficiency and safety.
Consider the chaotic world of finance, where market volatility can turn the tide overnight. With the influx of AI for fraud detection and real-time monitoring, institutions can transcend the traditional boundaries of risk management and respond swiftly to emerging threats. The liquidity of market conditions often necessitates the adoption of best predictive analytics tools for banking, which leverage credit scoring algorithms, default prediction models, and regulatory capital optimization strategies. These tools allow organizations to remain resilient and adaptable amidst challenges such as geopolitical risk and unpredictable economic fluctuations.
Moreover, organizations can now employ sophisticated stress testing techniques and scenario planning to address a spectrum of potential disturbances. Utilizing market volatility models allows businesses to simulate various conditions, making it easier to identify critical weaknesses. Predictive risk analytics not only enhances fraud pattern recognition but also enhances portfolio diversification strategies that protect against unforeseen events.
In essence, predictive risk analytics, underpinned by artificial intelligence, is more than just a tool; it is a vital component of modern risk management frameworks. As businesses strive to stay ahead of the competition, embracing these advanced techniques will be paramount in navigating the complexities of today’s risk environment, ensuring they remain prepared for whatever challenges lie ahead.
The Role of AI in Risk Management
Artificial intelligence (AI) is profoundly transforming the landscape of risk management, moving enterprises away from traditional methods towards more sophisticated, AI-driven approaches. This transition is akin to switching from a bicycle to a high-speed train; while both can serve the purpose of transportation, the latter offers a far more efficient and precise experience. As organizations grapple with increasing market volatility and complex geopolitical risks, predictive risk analytics powered by AI have emerged as invaluable tools for navigating these challenges.
One of the key advantages of incorporating AI into risk management is its automation capabilities. Tasks like default prediction and real-time monitoring, which once required significant time and manual effort, can now be performed swiftly and accurately. AI algorithms, including advanced credit scoring algorithms and fraud pattern recognition systems, analyze vast amounts of data in real-time, enabling financial institutions to detect and mitigate risks effectively before they escalate. Imagine having an assistant that not only reminds you of meetings but also actively predicts potential conflicts in your schedule — this is the transformative potential of AI in the realm of risk management.
Moreover, AI facilitates sophisticated stress testing and scenario planning, crucial for understanding the implications of various risks on an organization’s financial stability. By simulating different market conditions and examining the impact on portfolio diversification, firms can develop better response strategies. The best predictive analytics tools for banking harness AI to refine these models, ensuring they are not only accurate but also adaptable to changing economic climates.
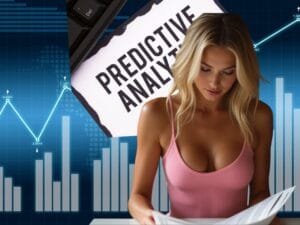
As organizations continue to embrace AI, the distinctions between conventional risk management strategies and modern, AI-enhanced methodologies become increasingly clear. The ability to leverage machine learning for automatic adjustments to risk profiles significantly enhances an institution’s regulatory capital optimization processes, making risk management not only more efficient but also more resilient. Ultimately, the integration of AI into risk management exemplifies the evolution from reactive to proactive strategies in an era characterized by uncertainty.
How AI is Transforming Risk Management
The landscape of risk management has undergone a substantial transformation attributed to the advent of artificial intelligence (AI). One of the most prominent changes is the introduction of predictive risk analytics, which leverages AI techniques to offer deeper insights into potential risks that organizations face. Utilizing advanced algorithms, firms can analyze vast amounts of data quickly and accurately, enabling them to anticipate market volatility more effectively.
For instance, the implementation of credit scoring algorithms incorporating AI allows financial institutions to assess the creditworthiness of individuals and businesses with greater precision. Unlike traditional scoring methods, these algorithms employ machine learning to adapt to new information, taking into account broader data sets that enhance the accuracy of default prediction. This not only results in improved decision-making but also promotes regulatory capital optimization by reducing the risk of non-performing loans.
Moreover, AI for fraud detection has revolutionized how organizations combat financial crimes. By employing intricate fraud pattern recognition techniques, banks can analyze transaction behaviors in real-time monitoring systems, identifying unusual activities that may indicate fraudulent behavior. This proactive approach leads to quicker intervention and reduced financial losses, further reinforcing trust between customers and financial institutions.
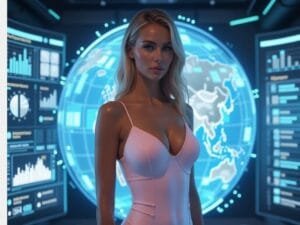
In addition to fraud detection, AI tools facilitate stress testing, enabling banks to simulate various economic scenarios. Through scenario planning, organizations can prepare for geopolitical risks and potential economic downturns, enhancing their resilience against unexpected market shifts. Portfolio diversification is another area where AI demonstrates noteworthy impact, as it helps financial managers make informed choices based on sophisticated market volatility models, ensuring that investment strategies are robust even in turbulent times.
AI continues to reshape the methods employed in risk management, incorporating innovative tools and techniques that empower organizations to respond adeptly to emerging threats while fostering sustainable growth.
Best Predictive Analytics Tools for Banking
The landscape of banking is rapidly evolving, and with it, the necessity for robust predictive risk analytics tools is becoming increasingly fundamental. These tools not only facilitate improved risk assessment but also allow financial institutions to maintain a competitive edge. By integrating artificial intelligence (AI) and machine learning, banks can enhance their risk management strategies and operational efficiencies. Here, we will explore some of the leading tools that are redefining the approach to risk management.
One notable option is SAS Visual Analytics. This tool offers advanced data visualization and interactive reporting features, making it accessible for stakeholders at various levels within an institution. By leveraging AI for fraud detection and credit scoring algorithms, it provides substantial insights, aiding in real-time monitoring and proactive decision-making.
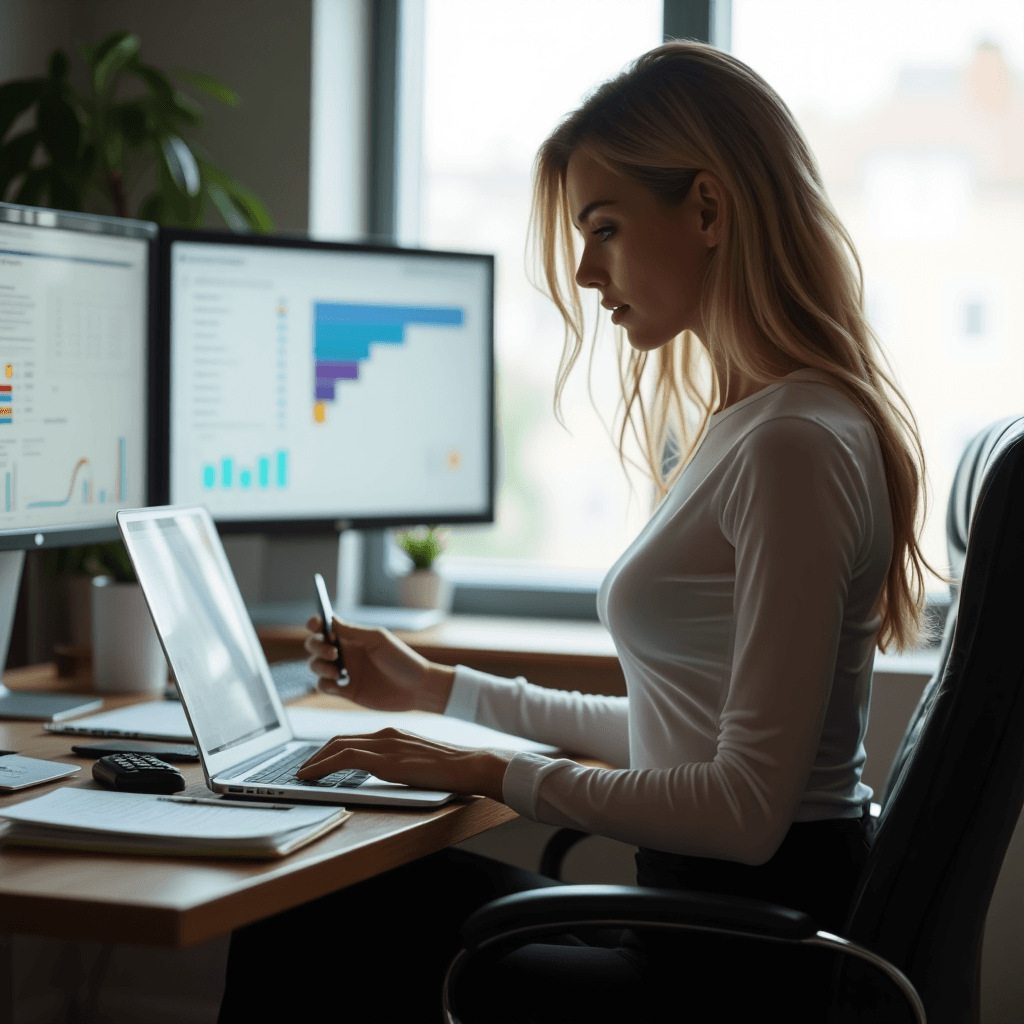
Another significant player is IBM Watson Analytics, renowned for its sophisticated natural language processing capabilities. This technology helps banks easily interpret vast datasets, allowing for better scenario planning amid market volatility. Furthermore, it enhances portfolio diversification strategies by analyzing historical trends and predicting future performance effectively.
FICO’s Xperience is also among the best predictive analytics tools for banking, specializing in predictive scoring models, particularly for default prediction. With strong capabilities in fraud pattern recognition, this tool enables institutions to identify suspicious activities swiftly, minimizing potential losses.
Lastly, Oracle Financial Services Analytical Applications provides extensive stress testing functionalities and regulatory capital optimization tools. Its robust architecture supports banks in navigating geopolitical risk while ensuring compliance with evolving regulations. The integration of these analytics tools simplifies risk management efforts, making banking processes not only safer but also more efficient. As the industry adapts to new challenges, leveraging these advanced predictive analytics tools remains essential for future success.
Key Concepts in Predictive Risk Analytics
Predictive risk analytics has emerged as a pivotal tool in risk management, primarily driven by advancements in artificial intelligence. This methodology involves forecasting potential risks and mitigating them effectively, thus enabling organizations to navigate the turbulent waters of financial volatility. Understanding the key concepts associated with predictive risk analytics is essential for leveraging its full potential.
One of the fundamental components is stress testing. This technique involves simulating extreme conditions to assess how financial portfolios might react during periods of market turmoil. Think of it as a workout for your financial strategies; just like lifting heavy weights can prepare your muscles for physical stress, stress testing your investments can fortify them against unforeseen events.
Equally important are market volatility models, which help measure how unpredictable the markets can be. These models assess various factors, including economic indicators and geopolitical risks, allowing organizations to prepare for sudden shifts. It’s akin to having a storm preparedness kit; you may never really need it, but it’s better to be safe than sorry when dark clouds gather on the horizon.
Scenario planning further enhances predictive risk analytics by allowing organizations to visualize potential future events based on different assumptions. This practice helps businesses strategize their responses proactively, whether they face new regulations or unexpected market events. By considering various scenarios, organizations position themselves to adapt quickly, minimizing potential risks and optimizing their regulatory capital.
In summary, mastering these key concepts—stress testing, market volatility models, and scenario planning—enables organizations to implement effective predictive risk analytics. This fundamental understanding lays the groundwork for enhancing fraud detection through AI, optimizing credit scoring algorithms, and refining default prediction methodologies, thereby transforming risk management into a proactive rather than reactive practice.
AI for Fraud Detection: A Digital Detective
In the ever-evolving landscape of finance and banking, fraud detection has become a paramount concern. The integration of artificial intelligence (AI) in this realm has acted like a digital detective, continuously on the hunt for smart criminals. AI systems utilize sophisticated algorithms and predictive risk analytics to recognize patterns that may indicate fraudulent activities. By sifting through vast amounts of data at incredible speeds, these tools can identify anomalies often overlooked by human analysts.

AI’s capability for fraud pattern recognition is particularly well-suited for real-time monitoring of transactions. For instance, credit scoring algorithms can be enhanced by leveraging AI to analyze historical data, potentially identifying fraudulent behaviors before they inflict damage. The AI algorithms analyze variables such as transaction frequency, geographic location, and user behavior, effectively allowing financial institutions to predict and mitigate risks associated with fraud.
One real-world application worth noting occurred in the banking sector, where AI systems identified an unusual spike in transactions tied to a single account. By applying market volatility models and stress testing, banks were able to set up alerts for any suspicious behavior emanating from this account, effectively stopping fraudulent actions in their tracks. The rapid decision-making capabilities of AI tools represent a transformative shift in risk management practices.
Moreover, scenario planning enabled through AI applications allows banks to simulate different fraud scenarios, enhancing their preparedness for potential threats. Such proactive approaches are critical as geopolitical risks also play a role in fraud schemes, and understanding these dynamics is essential for robust fraud prevention.
As financial institutions continue to deploy AI for fraud detection, they are not just enhancing security; they are optimizing regulatory capital and promoting confidence in the banking system. By harnessing the power of AI, organizations are revolutionizing their strategies, making it increasingly difficult for fraudsters to exploit vulnerabilities.
Challenges of Implementing AI in Risk Management
As organizations increasingly recognize the potential of AI in transforming predictive risk analytics, they encounter various challenges that can complicate the integration process. One of the most significant of these challenges is regulatory compliance. Financial institutions operate within a maze of regulations designed to protect consumer interests and maintain market integrity. Navigating this complex environment requires a keen understanding of how AI outputs align with regulatory frameworks. For instance, when deploying AI for fraud detection, institutions must ensure that their algorithms adhere to standards that safeguard against bias and discrimination. This level of diligence can slow down the adoption of advanced technologies.
Data integrity also poses a considerable hurdle. Effective AI models depend on high-quality, accurate data for training. If the input data is flawed or biased, the predictive analytics produced will also reflect these deficiencies, potentially leading to misguided risk assessments. Organizations must invest significant resources in cleaning and validating their data before feeding it into AI systems. Imagine attempting to bake a cake with expired ingredients; no matter how advanced your oven is, the outcome will likely be disappointing. The same principle applies to the realm of predictive risk analytics.
Furthermore, market volatility models can be sensitive to changes in input parameters. As global economic conditions fluctuate and geopolitical risks escalate, organizations must recalibrate their models continuously. This not only requires sophisticated technical expertise but also a commitment to real-time monitoring and scenario planning. It’s a dynamic but necessary approach to ensure robust risk management practices. Finally, the integration of AI into risk management demands a cultural shift within organizations. Employees may display apprehension about adopting AI tools, fearing job displacement or a lack of understanding. Fostering an environment of continual learning and adaptability is crucial to overcoming these challenges.
The Future of Predictive Risk Analytics
The landscape of predictive risk analytics is undergoing a significant transformation, largely due to the advancements in artificial intelligence (AI). Looking ahead, one can envision a future where AI technologies revolutionize risk management practices, particularly through enhancements in various sectors, including banking and finance. As institutions increasingly adopt AI for fraud detection and credit scoring algorithms, the efficacy of predictive risk analytics will undoubtedly improve, allowing organizations to identify vulnerabilities and mitigate risks more effectively.
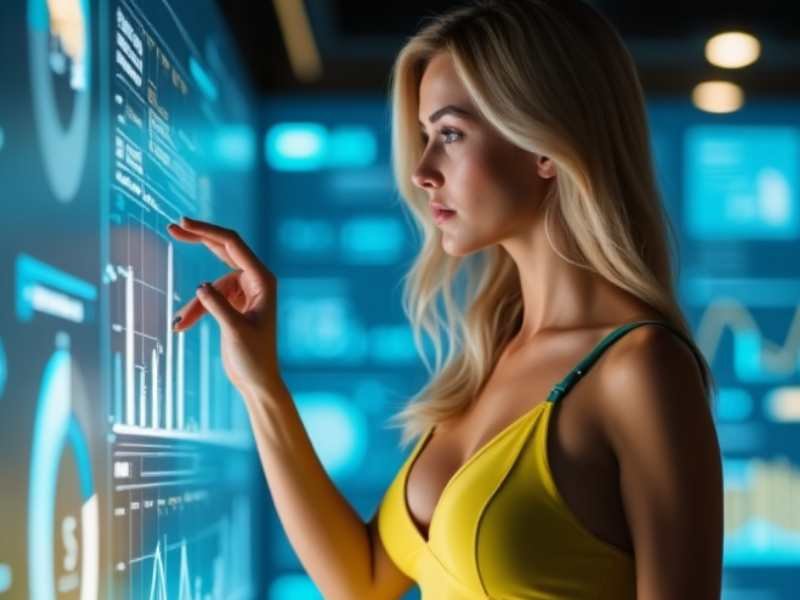
One compelling aspect of this transformation is the ability of AI to perform real-time monitoring and fraud pattern recognition. The immediacy and accuracy with which AI systems can analyze vast datasets lead to better decision-making and more robust risk mitigation strategies. This immediacy is crucial, especially in an environment characterized by market volatility, where timely insights can make the difference between profit and loss. Furthermore, as predictive analytics tools evolve, we can anticipate sophisticated market volatility models capable of forecasting downturns and enabling more effective portfolio diversification.
Moreover, the application of scenario planning will become increasingly refined as AI enhances our understanding of geopolitical risks and their implications. Institutions will be able to simulate various scenarios with remarkable accuracy, helping them prepare for potential disruptions and uncertainties. Stress testing models will transform as well, evolving to incorporate dynamic data feeds that allow for more relevant assessments of risk exposure in a rapidly changing world.
However, challenges remain that could impede this positive trajectory. Data privacy regulations, ethical considerations, and the need for interpretability in AI systems pose significant hurdles that the industry must address. By adequately navigating these challenges, the future of predictive risk analytics promises not only to enhance risk management processes but also to usher in a new era of proactive strategies that safeguard against potential threats. Maintaining an optimistic outlook is essential, as the fusion of AI with predictive analytics heralds unprecedented opportunities.
The Strategic Impact of AI on Predictive Risk Analytics
As we have explored throughout this blog post, the integration of artificial intelligence (AI) within risk management practices marks a transformative leap in predictive risk analytics. AI has not only enhanced the capabilities of risk assessment but has also streamlined processes such as fraud detection and credit scoring algorithms. By utilizing advanced predictive analytics tools for banking, organizations can improve their decision-making processes and optimize risk mitigation strategies.
Advanced Tools Empowering Real-Time Risk Response
Furthermore, innovations like market volatility models and stress testing have become increasingly sophisticated, allowing institutions to perform scenario planning more effectively. The application of AI for real-time monitoring facilitates immediate responses to fluctuating conditions and geopolitical risks, enabling businesses to navigate complex landscapes with greater confidence. With tools that enhance fraud pattern recognition and default prediction, institutions are better equipped to protect assets and ensure regulatory compliance.
The Evolving Future of AI-Driven Risk Management
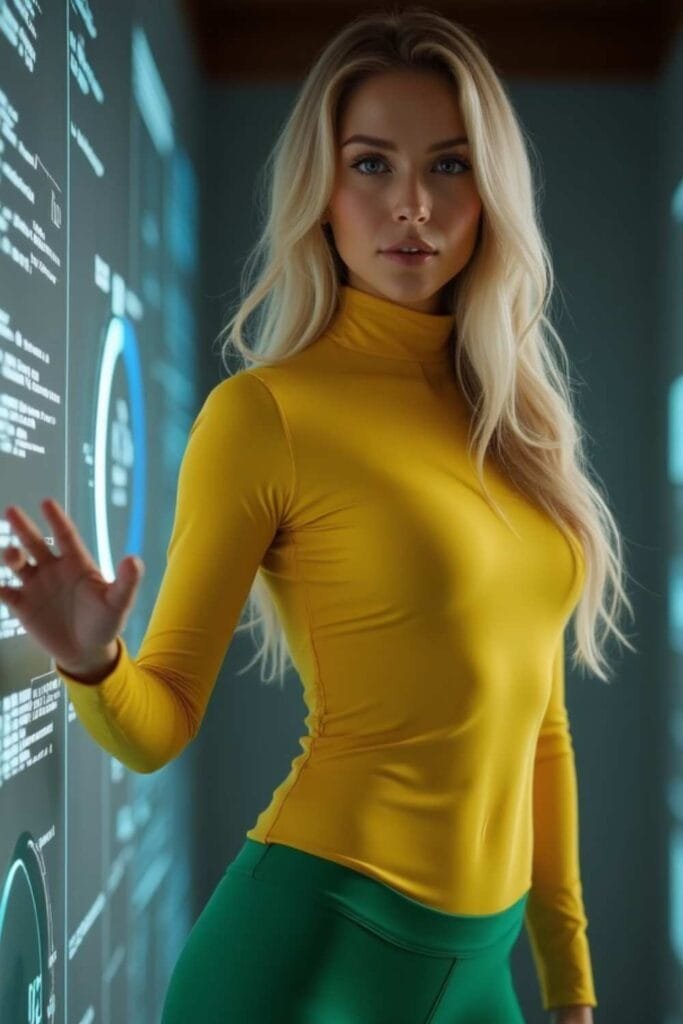
It is essential to recognize that the journey of AI in risk management is ongoing. Continuous improvements in predictive risk analytics will likely lead to enhanced regulatory capital optimization and successful portfolio diversification. As businesses increasingly adopt AI technologies, the potential for more accurate risk assessments and superior management strategies will continue to expand.
Join the Conversation: How Is AI Shaping Your Risk Strategy?
We invite you to share your thoughts and experiences related to the use of AI in risk management. How do you think predictive risk analytics has changed the landscape of your industry? Have you utilized any of the discussed tools or strategies in your organization? Let’s engage in a meaningful conversation about the future of AI in risk management and explore how we can collectively navigate this evolving field.